Yihua Zhang
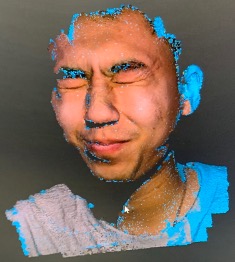
Room 3210
428 S Shaw LN
East Lansing, Michigan
United States of America
I am Yihua Zhang (张逸骅), a third-year Ph.D. student from OPTML Group at Michigan State University, supervised by Prof. Sijia Liu. My research focuses on the trustworthy and scalable ML algorithms. In general, my research spans the areas of machine learning (ML)/deep learning (DL), optimization theory, computer vision, and security. These research topics provide a solid foundation for my current and future research: Making AI system responsible and efficient. My research on these two goals are intervened and can be summarized as the following two perspectives:
Algorithmic perspective: This line of research designs the scalable and theoretically-grounded machine learning algorithms subject to real-life constraints, including bi-level optimization, zeroth-order optimization, inviriant risk minimization, etc.
Application perspective: This line of research tackles the domain-specific challenges to achieve scalable and trustworthy AI, including data and model pruning, efficient model structures, model robustness and unlearning, etc.
News
Jul 1, 2024 |
![]() |
---|---|
May 15, 2024 |
![]() |
May 1, 2024 |
![]() |
Feb 18, 2024 |
![]() |
Feb 14, 2024 |
![]() |